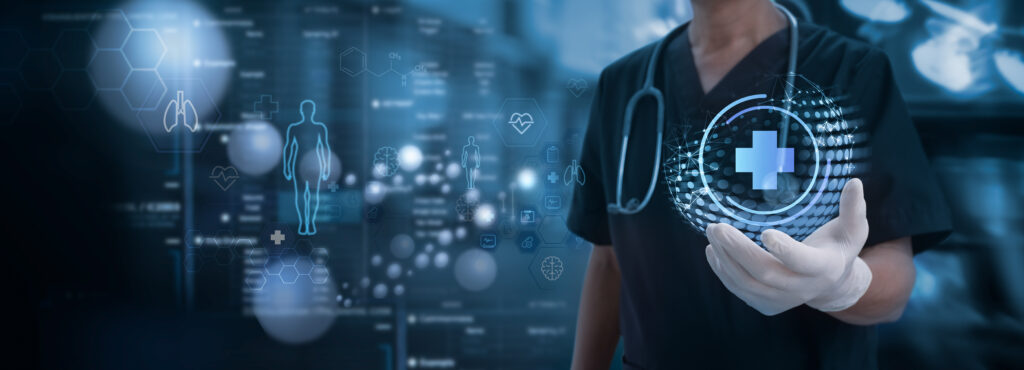
Navigating the Path to Strategic AI Implementation in Healthcare
The integration of artificial intelligence (AI) into healthcare holds the promise of revolutionizing patient care, offering more personalized, precise treatment options, and improving overall health outcomes. From predictive analytics and early diagnosis to personalized treatment plans and operational efficiency, AI has the potential to transform every aspect of the healthcare industry. However, the road to fully realizing AI’s potential is fraught with challenges, including those related to data quality, algorithmic trust, skill gaps, patient-centricity, and regulatory compliance. Overcoming these obstacles requires a strategic approach that balances innovation with the practical realities of clinical settings.
Data Quality and Bias: The Foundation of Effective AI
AI’s effectiveness is intrinsically tied to the quality of data it processes. In healthcare, data is often siloed, unstructured, or incomplete, leading to potential inaccuracies in AI-driven insights. Moreover, data bias—whether due to underrepresentation of certain demographic groups or inherent flaws in data collection methods—can skew AI models, perpetuating health disparities rather than alleviating them.
To tackle these issues, healthcare organizations must prioritize robust data governance. This involves implementing comprehensive data management strategies that ensure data is accurate, up-to-date, and representative of diverse populations. Routine audits, standardization of data formats, and collaboration across institutions can help maintain data integrity. Additionally, addressing bias requires a proactive approach, including the development of bias-detection mechanisms and the continuous evaluation of AI outputs to ensure fairness and equity in healthcare delivery.
Building Algorithmic Trust: The Key to Adoption
Trust is a cornerstone of AI adoption in healthcare. Without it, clinicians and patients alike may resist relying on AI-driven decisions, regardless of the technology’s potential benefits. Building this trust starts with ensuring that AI algorithms are transparent, explainable, and subject to rigorous validation.
Transparency involves making AI processes understandable to non-technical stakeholders. Explainable AI (XAI) techniques can help by breaking down complex algorithmic decisions into interpretable components, allowing clinicians to see how and why a particular recommendation was made. This is particularly important in high-stakes environments like healthcare, where decisions can have life-or-death consequences.
Validation of AI models is equally critical. Before deployment, AI tools must undergo extensive testing in real-world settings to ensure their accuracy and reliability. This includes comparing AI-generated outcomes with traditional methods and continuously monitoring performance to identify any emerging issues. By fostering transparency and ensuring robust validation, healthcare organizations can build the trust necessary for widespread AI adoption.
Addressing Skills Deficits: Preparing the Workforce
The successful implementation of AI in healthcare is not just a technological challenge—it’s also a human one. The healthcare workforce must be equipped with the skills and knowledge needed to effectively use AI tools. This requires a concerted effort to bridge the gap between healthcare professionals and AI technologies.
Education and training are key components of this effort. Healthcare providers need to be educated not only on the basics of AI but also on how to interpret AI-generated data and integrate AI tools into their daily practice. This can be achieved through targeted training programs, continuous professional development opportunities, and interdisciplinary collaboration between clinicians, data scientists, and AI specialists.
Moreover, fostering a culture of collaboration is essential. Encouraging partnerships between healthcare professionals and AI experts can lead to the co-creation of AI solutions that are both technically sound and clinically relevant. By addressing skills deficits and promoting collaboration, healthcare organizations can ensure that their workforce is ready to leverage AI to its fullest potential.
Ensuring Patient-Centricity: AI with the Patient in Mind
At the heart of healthcare is the patient. As such, any AI solution must be designed and implemented with a focus on patient-centricity. This means that AI tools should enhance patient care and experience, rather than simply driving operational efficiencies or cost reductions.
One way to ensure patient-centricity is to engage patients in the AI development process. This can involve seeking patient input on the design and functionality of AI tools, as well as involving them in decision-making processes regarding AI-driven care. Additionally, AI solutions should be designed to support personalized care, taking into account individual patient preferences, needs, and values.
Furthermore, patient data privacy must be a top priority. With the increasing use of AI, the volume of patient data being collected, stored, and analyzed is growing. Healthcare organizations must implement stringent data security measures to protect patient information and maintain trust. By keeping patient-centricity at the forefront, healthcare organizations can develop AI solutions that truly meet the needs of those they serve.
Navigating Regulatory Compliance: Balancing Innovation and Safety
The healthcare industry is heavily regulated, and for good reason—patient safety and well-being are paramount. However, the introduction of AI into healthcare introduces new regulatory challenges. AI tools must comply with a range of regulations, including those related to patient privacy, safety, and efficacy. Navigating this regulatory landscape requires a careful balance between fostering innovation and ensuring compliance.
Regulatory bodies are still adapting to the rapid pace of AI innovation, leading to a sometimes unclear regulatory environment. Healthcare organizations must stay informed about evolving regulations and engage with regulatory bodies to ensure their AI solutions meet all necessary standards. This includes obtaining proper certifications, adhering to data protection laws, and ensuring that AI tools are rigorously tested before deployment.
Moreover, leaders in healthcare should advocate for policies that support the ethical and safe use of AI, while also pushing for regulatory frameworks that enable innovation. By actively participating in the regulatory process, healthcare organizations can help shape a future where AI contributes positively to patient care without compromising safety or ethics.
Conclusion: A Strategic Approach to AI in Healthcare
The potential of AI to transform healthcare is undeniable. However, the journey from potential to reality is complex, requiring a strategic approach that addresses the unique challenges of the healthcare environment. By focusing on data quality and bias, building algorithmic trust, addressing skills deficits, ensuring patient-centricity, and navigating regulatory compliance, healthcare leaders can successfully implement AI solutions that improve patient outcomes, enhance the quality of care, and drive innovation in the industry.
The key to success lies in balancing technological advancements with the human elements of healthcare delivery. With a thoughtful, patient-centered approach, AI can become a powerful tool in the healthcare arsenal, helping to tackle modern challenges and pave the way for a healthier future.